Machine learning applications in computational biology
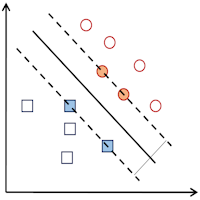
We apply conventional and deep machine learning to data analysis problems in proteomics and other high throughput data fields. On the one hand we utilize the flexibility and predictive strength of deep learning models, such as recurrent and convolutional neural networks. On the other hand, we are Interested In finding alternatives from conventional machine learning in time-critical applications, that reach similar predictive performance In much less computing time.
We developed the DeepMass:Prism deep learning model for the prediction of MS/MS fragmentation spectra based on the peptide sequence, which was published as an article in Nature Methods. It is the basis for further research in my lab to integrate and improve peptide identification in data-dependent and data-independent proteomics.
We currently work on de-novo identification of peptides in DDA and DIA data. We are generating machine learning models for the prediction of several other peptide properties from sequence to aid the identification process. Also, we apply machine learning for the guidance of missing value imputation in proteomics data and for the prediction of mass-spectrometric properties of other molecules such as metabolites.
Selected publications:
Cox, J. and Mann, M. (2012) 1D and 2D annotation enrichment: A statistical method integrating quantitative proteomics with complementary high-throughput data. BMC Bioinformatics, 13 Suppl 16:S12.
Robles, M.S., Cox, J. and Mann, M. (2014) In-vivo quantitative proteomics reveals a key contribution of post-transcriptional mechanisms to the circadian regulation of liver metabolism. PLoS Genetics 10(1):e1004047.
Geiger, T., Cox, J. and Mann, M. (2010). Proteomic changes resulting from gene copy number variations in cancer cells. PLoS Genetics 6.