Personalized Medicine
Machine Learning for Personalized Medicine | Personalized Swiss Sepsis Study | Machine Learning Frontiers in Precision Medicine |
Why do some persons suffer from diabetes or Alzheimer's disease? Why does a cancer treatment work well for some patients but for others not at all? And why do some patients suffer from adverse effects of the treatment?
The answers to these and similar questions is: All persons have different genetic properties, and the genes affect if a person becomes sick or how medications take effect in the body.
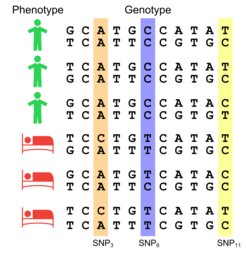
The main challenge is to find associations between the genotype and the phenotype (e.g., the onset or course of a disease, the effect of a treatment). As the human genome consists of billions of bases, gigantic amounts of data have to be analysed. Here machine learning comes into play.
We develop efficient algorithms to detect patterns, rules and statistical dependencies in large medical datasets with the ultimate goal to improve healthcare in collaborations with medical researchers.
We have been involved in several national and international networks and collaborations on Personalized Medicine:
- Coordinating node of the MSCA Initial Training Network Machine Learning for Personalized Medicine (2013-2016)
- Coordinating node of the SPHN/PHRT Driver Project Personalized Swiss Sepsis Study (2018-2023)
- Coordinating node of the MSCA Initial Training Network Machine Learning Frontiers in Precision Medicine (2019-2022)
Machine Learning for Personalized Medicine

From 2013 to 2016, we coordinated the Marie Curie Initial Training Network on Machine Learning for Personalized Medicine (MLPM). The network consisted of several universities, research institutions and companies located in Spain, France, Germany, Belgium, UK, Switzerland, Israel and in the USA. MLPM trained 14 young scientists in the research field at the interface of Machine Learning and Medicine to become interdisciplinary experts who will develop and employ the computational and statistical tools that are necessary to enable personalized medical treatment of patients.
More information is available on the MLPM webpage: https://mlpm.eucall_made
This project has received funding from the European Union 7th Framework Program through the Marie Curie ITN MLPM grant No 316861. |
Personalized Swiss Sepsis Study
From 2018 to 2023, the Personalized Swiss Sepsis Study was a SPHN/PHRT driver project with the goal to build an interoperable infrastructure among the intensive care units of the Swiss university hospitals and several research groups, to gather complex information on the host and pathogen during the entire course of a sepsis. The integration of continuous monitoring data from intensive care units will result in digital biomarkers. Combined with the molecular data from bacterial pathogens (metagenomics and whole genome sequencing) and from the host (metabolomics, immunophenotyping, genotyping), new avenues for sepsis research will emerge. These very comprehensive and complex data will be combined via the SPHN data hubs to enable multi-dimensional analyses through machine learning. The goal is to recognize a bacterial sepsis earlier and to predict its course more precisely than currently possible for an individual patient.
Coordinators
Karsten Borgwardt (ETH Zürich)
Adrian Egli (University Hospital Basel)
Collaborators
ETH Zürich | Karsten Borgwardt (PI), Peter Bühlmann, Nicolai Meinshausen, Gunner Rätsch, Nicola Zamboni |
University Hospital Basel | Manuel Battegay, Adrian Egli (PI), Stephan Marsch, Martin Siegemund |
University of Basel | Christoph Dehio |
University Hospital Zürich | Reto Schüpbach, Annelies Zinkernagel |
University of Zürich | Reinhard Zbinden |
University Hospital Bern Insel | Hansjakob Furrer, Stephan Jakob |
University of Bern | Stephen Leib |
CHUV Lausanne | Thierry Calandra, Jean-Luc Pagani, Gilbert Greub |
University Hospital Geneva | Laurent Kaiser, Jerome Pugin, Jacques Schrenzel |
Publications
SPHN/PHRT: Forming a Swiss-Wide Infrastructure for Data-Driven Sepsis Research
Adrian Egli, Manuel Battegay, Andrea C Büchler, Peter Bühlmann, Thierry Calandra, Philippe Eckert, Hansjakob Furrer, Gilbert Greub, Stephan M Jakob, Laurent Kaiser, Stephen L Leib, Stephan Marsch, Nicolai Meinshausen, Jean-Luc Pagani, Jerome Pugin, Gunnar Rätsch, Jacques Schrenzel, Reto Schüpbach, Martin Siegemund, Nicola Zamboni, Reinhard Zbinden, Annelies Zinkernagel, and Karsten Borgwardt
Studies in Health Technology and Informatics 2020, 270: 1163–1167
Online
Early Recognition of Sepsis with Gaussian Process Temporal Convolutional Networks and Dynamic Time Warping
Michael Moor, Max Horn, Bastian Rieck, Damian Roqueiro and Karsten Borgwardt
Machine Learning for Healthcare Conference (MLHC) 2019 and Proceedings of Machine Learning Research 106:2–26, 2019
Online | GitHub | ETH Research Collection
This project is supported by the grant #2017‐110 of the Strategic Focal Area Personalized Health and Related Technologies (PHRT) of the ETH Domain and the Swiss Personalized Health Network (SPHN) (2017DRI20).
PSSS website: https://sepsis-network.ch
Machine Learning Frontiers in Precision Medicine
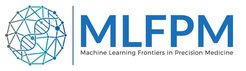
From 2019 to 2022, we coordinated the Marie Curie Innovative Training Network entitled "Machine Learning Frontiers in Precision Medicine" bringing together leading European research institutes in machine learning and statistical genetics, both from the private and public sector. We train 14 early stage researchers who will apply machine learning methods to health data. The goal is to reveal new insights into disease mechanisms and therapy outcomes and to exploit the findings for precision medicine, which hopes to offer personalized preventive care and therapy selection for each patient.
Network partners
The MLFPM network consists of the following partner institutions and PIs: ETH Zürich (Karsten Borgwardt, Caroline Uhler), University of Liege (Kristel Van Steen), Max-Planck-Gesellschaft zur Förderung der Wissenschaften e.V. (Bertram Müller-Myhsok, Bernhard Schölkopf and Gabriele Schweikert), Siemens Healthcare GmbH (Tobias Heimann and Volker Tresp), University of Tartu (Krista Fischer), STACC (Jaak Vilo), Fundación Pública Andaluza Progreso y Salud (Joaquin Dopazo), Universidad Carlos III de Madrid (Antonio Artés), ARMINES (Chloé-Agathe Azencott), Université Paris Descartes (Florence Demenais), Qlucore (Carl-Johan Ivarsson and Magnus Fontes), IBM Israel (Tal El-Hay and Michal Rosen-Zvi), Pharmatics (Felix Agakov) and Roche (Antonia Stank).
Funding
The total funding for MLFPM is EUR 3 638 275,56.
Two MLFPM fellows at MLCB lab
Two of the 14 Early Stage Researchers will conduct their work at the MLCB lab. ESR1 is working on "Machine Learning for Biological Network Analysis", and ESR2 is working on the project "Machine Learning and Causal Inference to Optimize Genomic Interventions using Disease State Representations".
More information is available on the MLFPM webpage: https://mlfpm.eu
This project has received funding from the European Union’s Horizon 2020 research and innovation programme under grant agreement No 813533. |